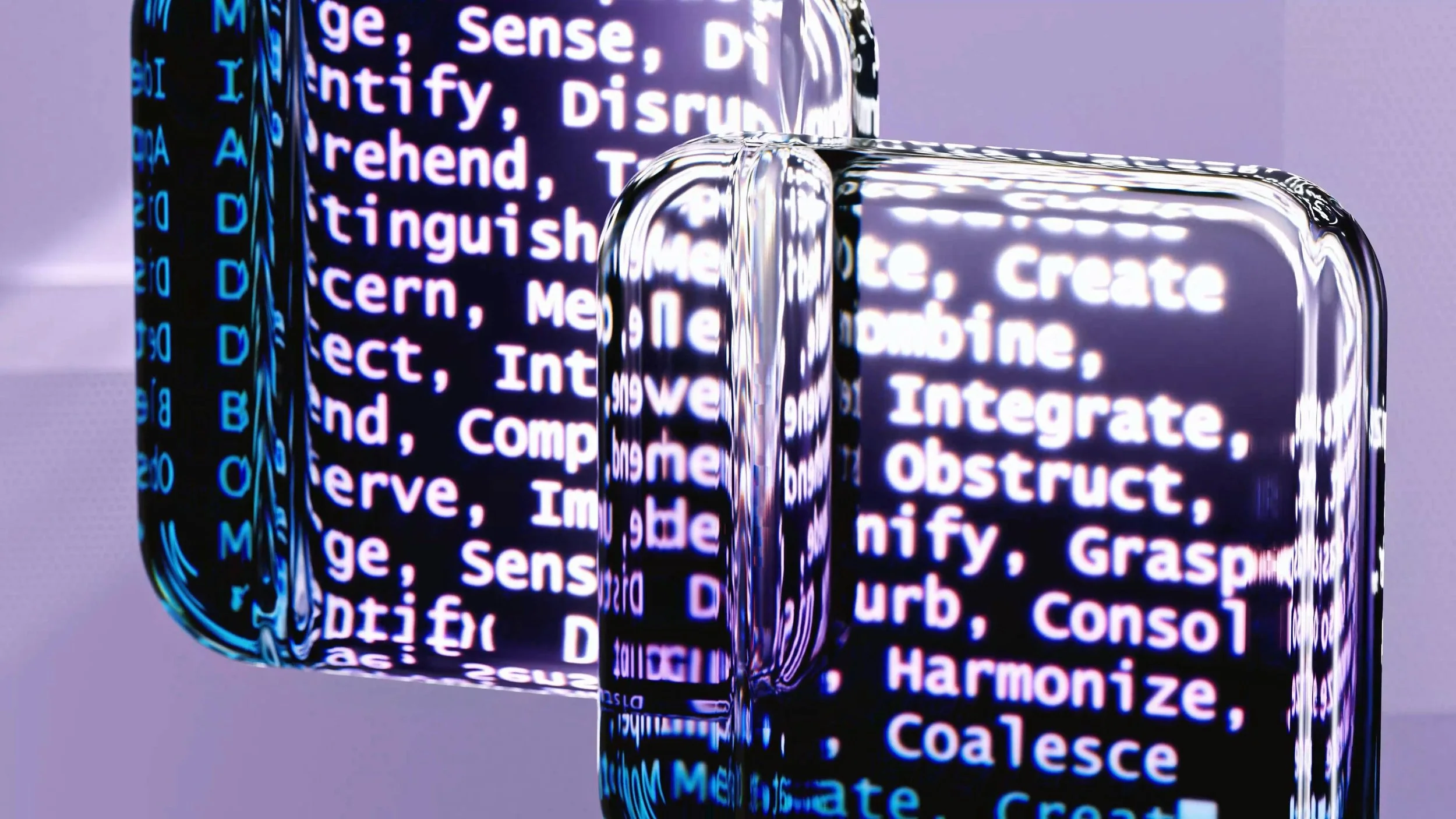
Azure AI Search: The “R” in Retrieval Augmented Generation
Retrieval-Augmented Generation (RAG) is a technique that significantly enhances AI’s ability to answer questions accurately by retrieving relevant information from external documents and integrating it into responses. The retrieval component serves as a bridge between AI and specific data sources, pulling in the most relevant, up-to-date information to address each question. By grounding AI-generated answers in precise, factual data, RAG overcomes the limitations of language models that may lack current or specialized information. High-quality retrieval is crucial for RAG to succeed, as it directly impacts the accuracy and relevance of the AI’s responses.

Unlocking Your Data: How LLMs Empower Businesses to Tap into Data Warehouses — Part 2 — In the Lab
In our previous discussion on Unlocking Your Data, we explored how Large Language Models (LLMs) can facilitate access to data warehouses, enabling non-technical users to derive valuable insights without requiring SQL knowledge or advanced technical skills. By removing barriers to data access, LLMs empower employees across all business functions, accelerating decision-making and driving data-driven innovation.

Unlocking Your Data: How LLMs Empower Businesses to Tap into Data Warehouses
In today’s data-driven world, organizations rely heavily on advanced data warehouses to store vast amounts of information. These platforms are essential for aggregating, analyzing, and leveraging data, but access to the insights they contain is often limited to technical users with expertise in SQL or specialized business intelligence tools like Power BI and Tableau. This creates a bottleneck, where non-technical users are unable to independently access databases to obtain insights, slowing down decision-making and limiting data-driven innovation.